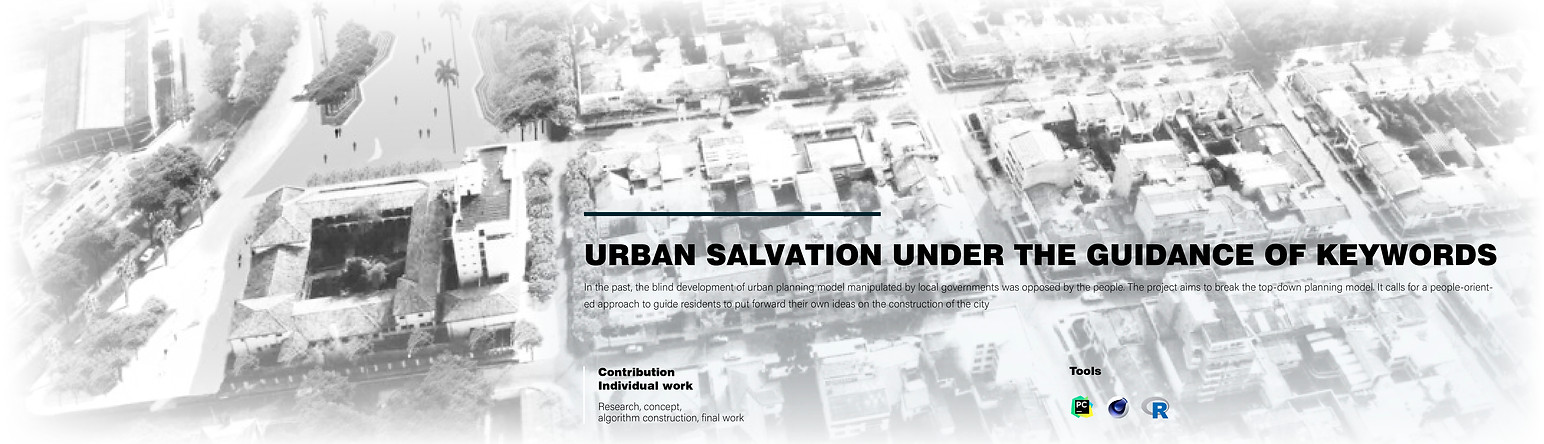
Urban Salvation
A people-centered project for predicting future cities
TIMELINE
Tools
Pycharm, C4D, R
''
Oct 2020-Feb 2021
PROJECT TYPE
Program Design
Contribution
Individual Work
Services
Research, Concept,
Algorithm construction,Final work
As an urban planner, social responsibility is undoubtedly one of the essential qualities. I select many images of possible future urban elements such as intelligent buildings, interactive streets, and different kinds of green plants, add the location information of target plots and obtain the final visualization results through deep learning model training.
I hope this project can assist with the planning and design. Residents can intuitively see the process and results of urban change under the guidance of keywords and express their thoughts.
What is the purpose of this project?
Background
Location Analysis
In order to better understand the essential information and situation of the site. I searched the information on this area and conducted field research. Finally, I summarized the results and formed a background mapping investigation of the Xuanwu historical block as below.
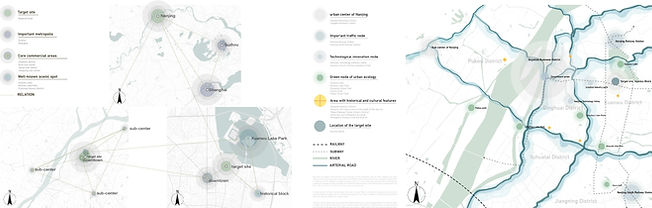
Historical Evolution Collage
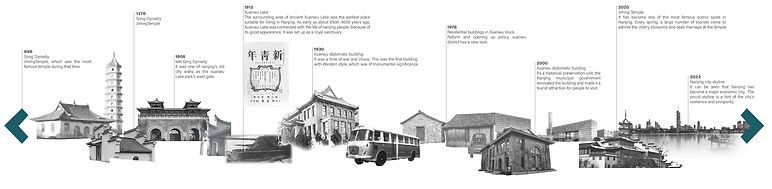
This region has a long history. Today, the local architecture is still a blend of ancient and modern scenes.
Comprehensive Analysis
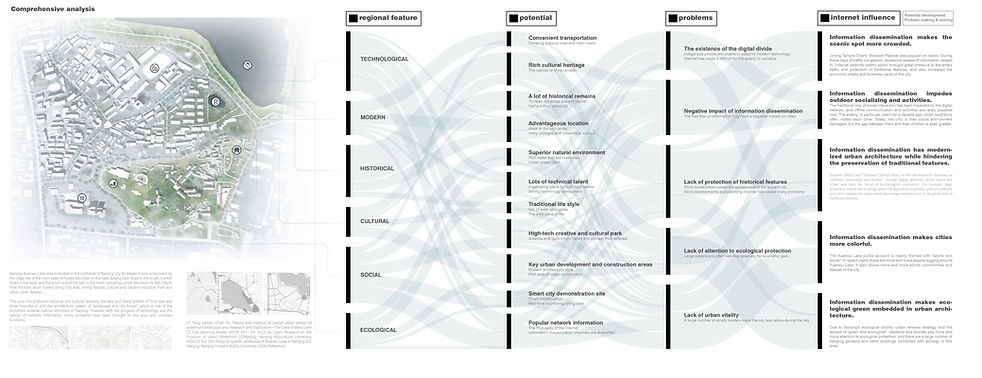
User Test
Method
Considering the influence of age and gender on the research results, I tend to select people of different age groups and different gender groups as objects to make the research results more convincing. Specifically, I will issue questionnaires and interview residents and tourists in the design base, inform them of the influence of information dissemination and ask them the words they most want to put in this area.
Location
No.1, Xuanwu Street, Xuanwu District, Nanjing, China
It is located in downtown Nanjing, with purple Mountain in the east and Jiuhua Mountain in the south.
Subject
Based on the various problems of this area and the influence of Internet information, we issued 169 questionnaires and selected 30 local men and women of different ages for interviews. Finally, I summarized the sentences and words they answered into 30 keywords that can be put into the area.


After the interview, I summarized the replies of 30 interviewees and translated their answers into one most appropriate word.
After that, I found potential connections between the words. I connected the words with a strong connection through the data line graph and finally concluded into seven word blocks. Surprisingly, these seven blocks correspond to the core problem of this area.

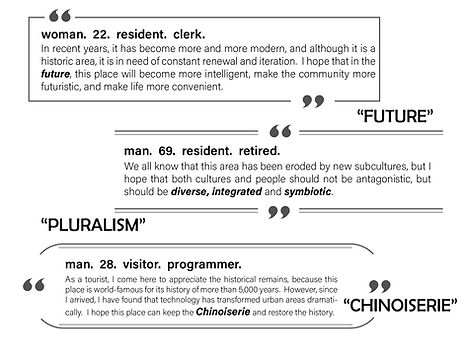
According to the survey, most residents are not satisfied with the city's current state. They are not content to live in the current construction environment. Most people believe that in today's urban planning system, the proportion of residents' opinions needs to be higher, resulting in cities that do not develop according to their wishes. Residents also believe that the area should now think in a people-oriented, technical direction.
Design Concept
Considering the problems in the target site and the rigid process of traditional urban planning, I plan to build a bottom-up planning system. Predicting future urban development driven by keywords is an integral part of the system. By visualizing future urban development direction, innovative planning ideas can be inspired to alleviate urban problems effectively.
Traditional Planning Process
Top-Down

Concept Generating

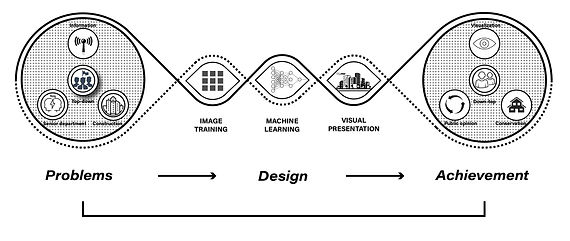
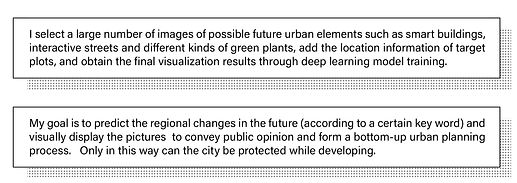
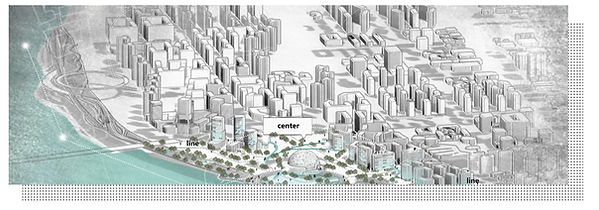
Improved Planning Process
Down-Top
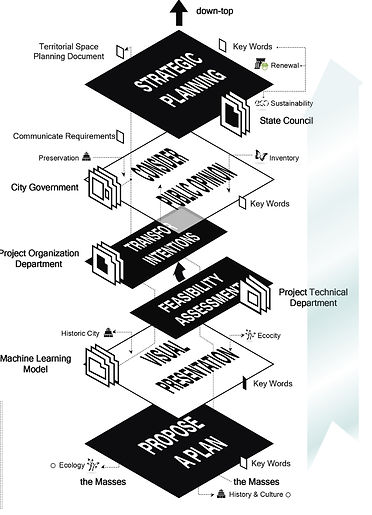
System Design
Introduction to GAN(Generative Adversarial Networks) & WGAN(Wasserstein GAN) models
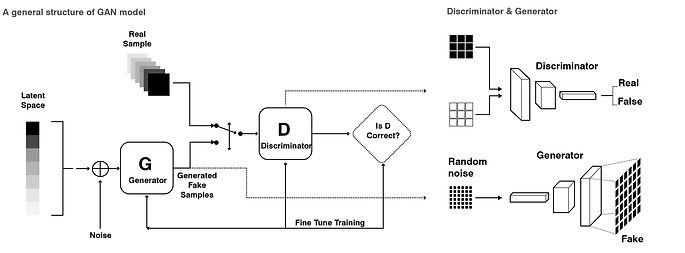
Open Problems
◆ GAN training is really unstable.
◆ There are collapse mode problems.
◆ The generated results are not rich enough.
◆ Poor interpretability, no explicit expression of distribution Pg(G) of the generated model.
◆ It is difficult for the network to converge.
Improvements
◆ WGAN theoretically gives the reason for the instability of GAN training. The cross-entropy (JS divergence) is unsuitable for measuring the distance between the distributions with disjoint parts. Wasserstein distance is used instead to measure the distance between the generated and accurate data distribution.
◆ Solved the collapse mode problem and generated more diversified results.
Overall Workflow
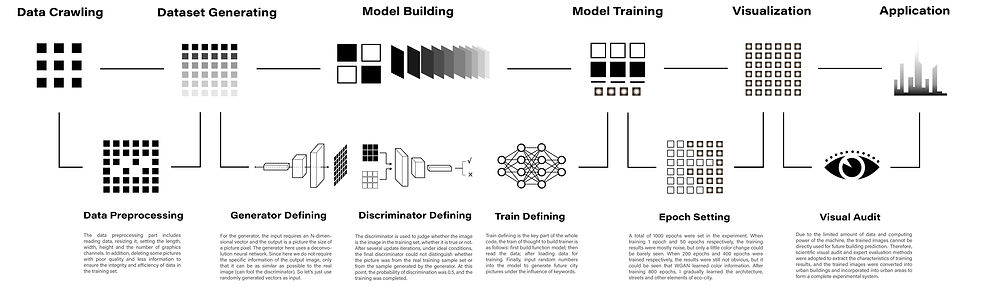
Training result
Deep Learning Process
Keyword: Technological
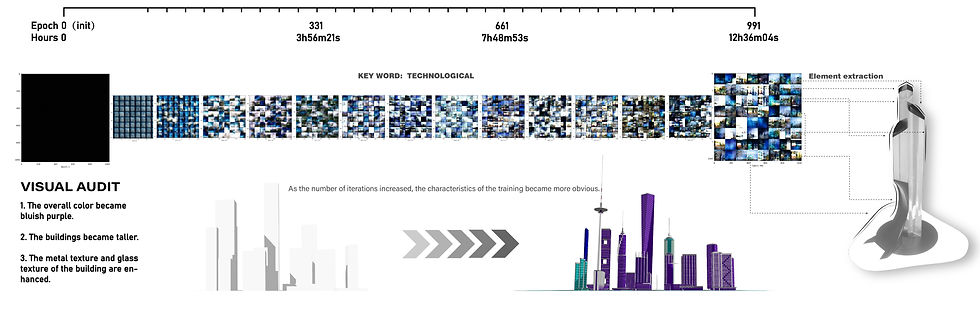
Deep Learning Process
Keyword: Ecological
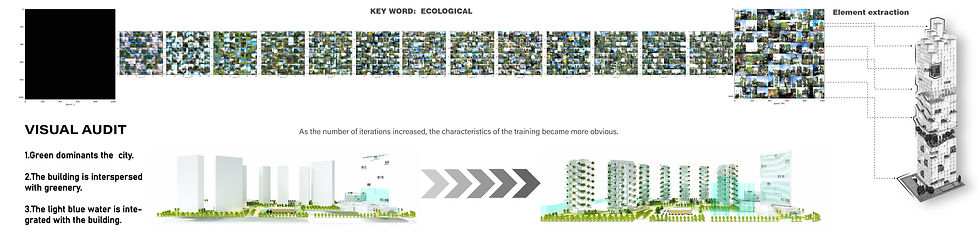
Web Design
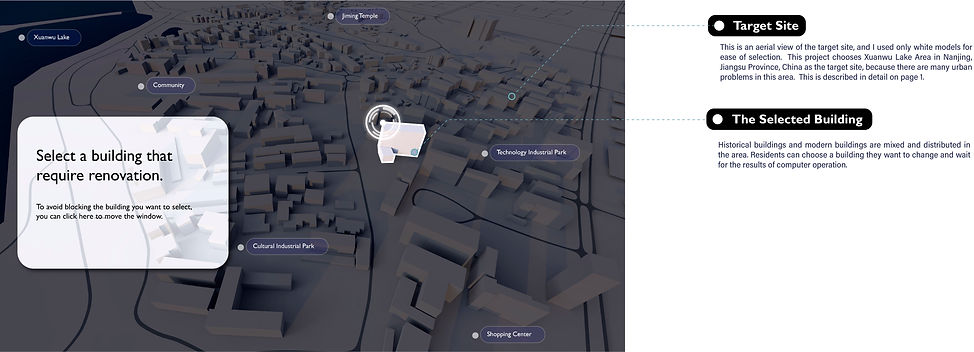
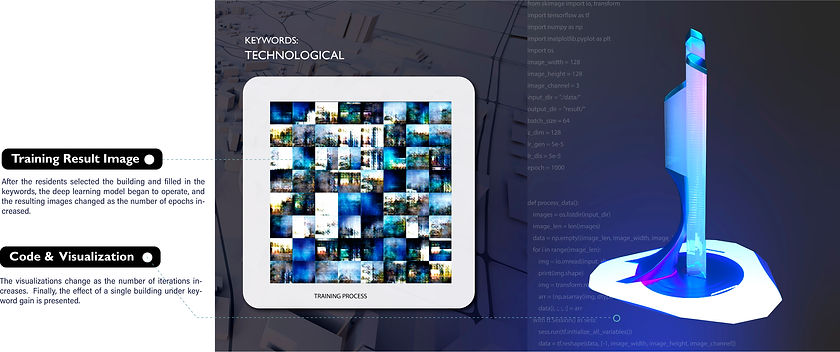
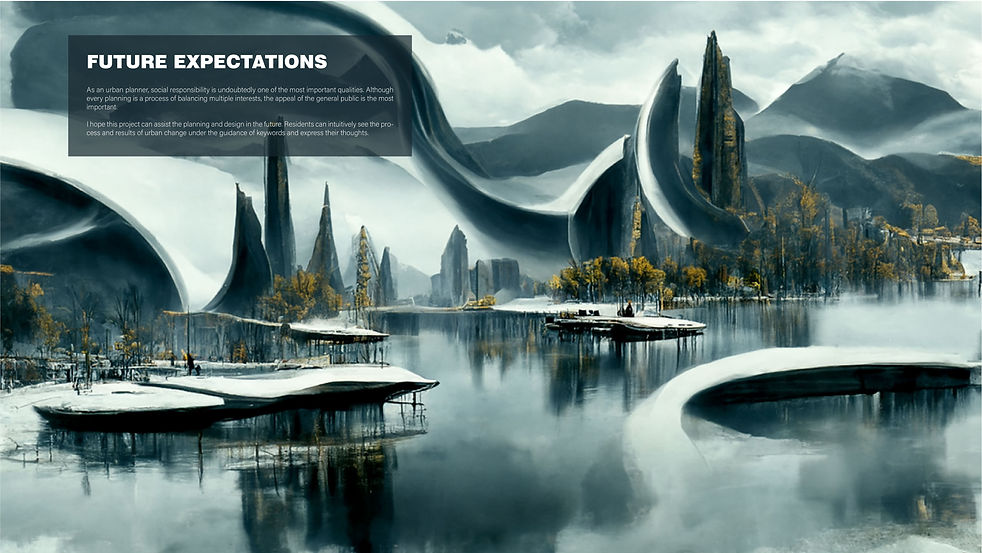